
Are you interested in bringing artificial intelligence and data science to the forefront of health research? Three top-level institutions – the Karlsruhe Institute of Technology, the German Cancer Research Center and Heidelberg University – have joined forces to tackle this exciting area by providing a unique doctoral program. Send your application if you can see the potential in techniques such as deep learning in the health sector and if you are ready to shape the future of our well-being.
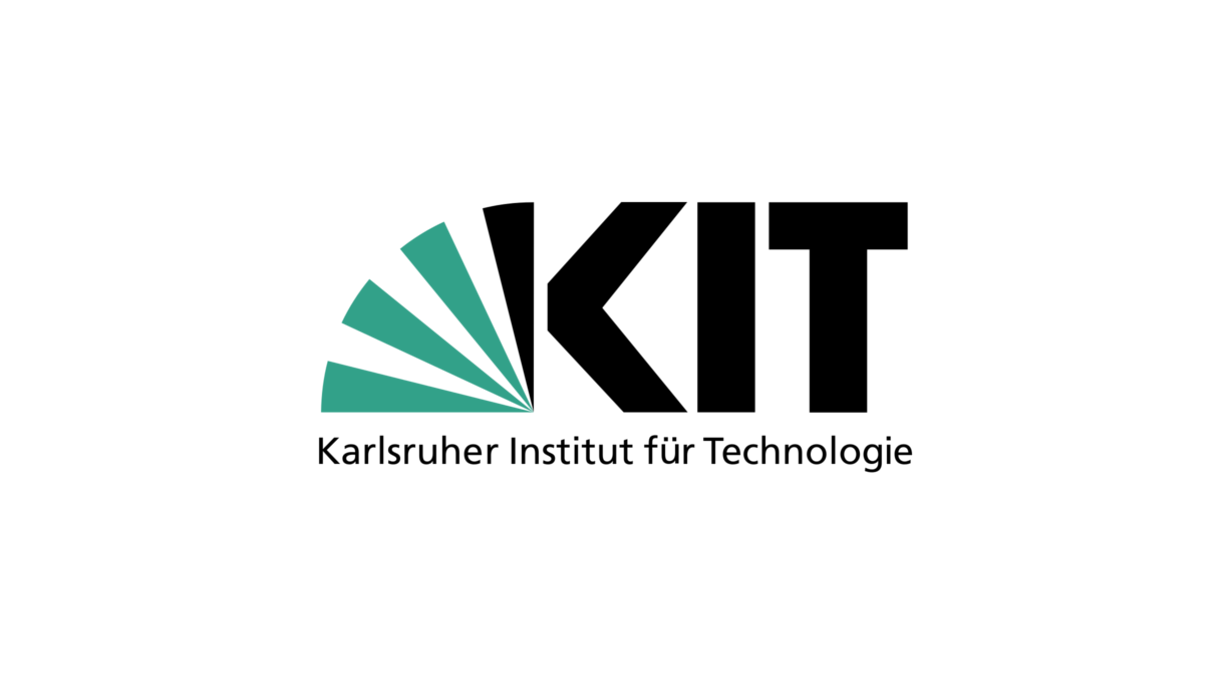
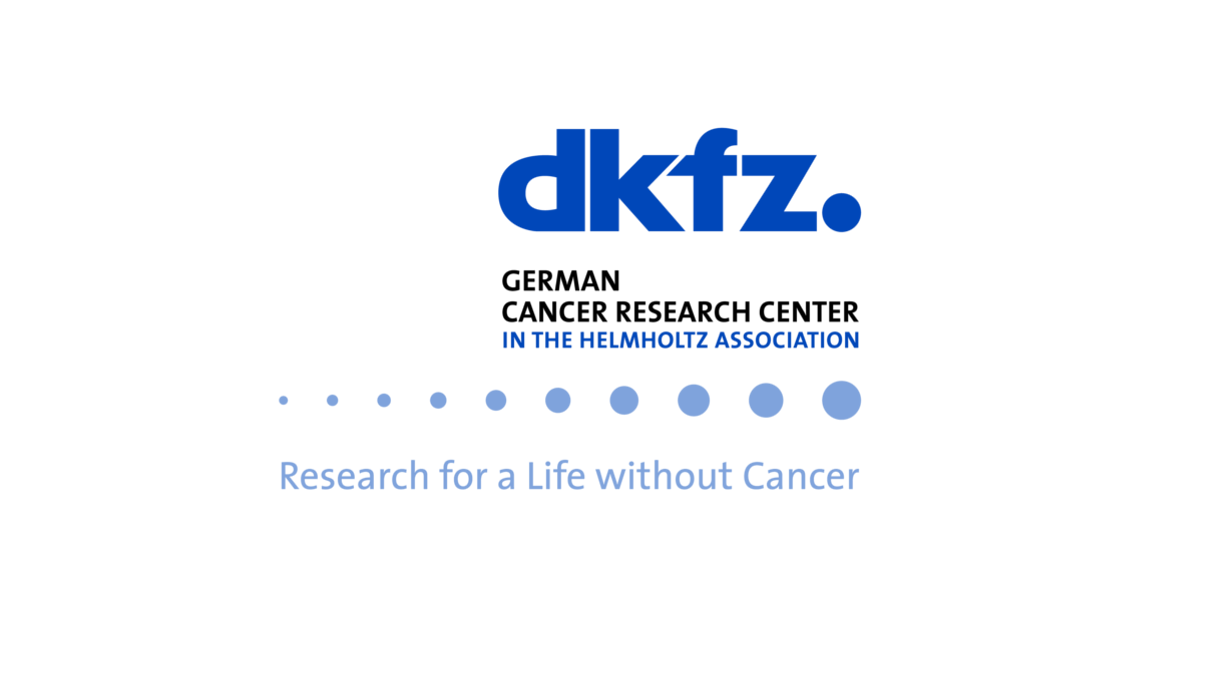
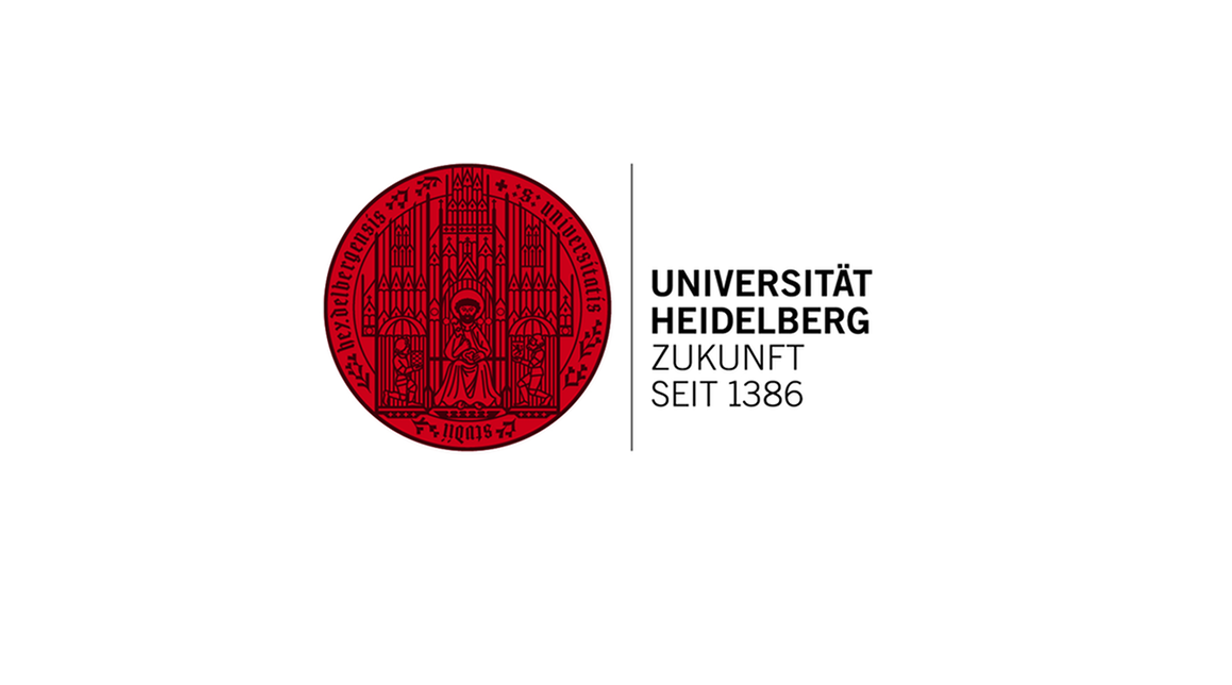

Mission Statement
The aim of the Helmholtz Information & Data Science School for Health (HIDSS4Health) is to attract, promote and train the best young talents at the interface between data science and health-related applications. HIDSS4Health offers a structured doctoral training program embedded in a highly interdisciplinary research environment, bringing together experts from the data and life sciences. The scientific curriculum is complemented by training measures that provide doctoral researchers with the key qualifications expected from future leaders in science and industry.

Research Areas




Projects
Projects 2022 (see also the proposals)
Imaging & Diagnostics

While there has been impressive progress in the field of biomedical image segmentation, current approaches hardly incorporate anatomical knowledge or common... (read more)

Organoids are self-assembled three-dimensional aggregates generated from human pluripotent stem cells (hPSC) with cell types and cytoarchitectures that resemble human organs... (read more)

Current image analysis of patient data only uses single images, with previous measurements not being incorporated into the model. Furthermore, image... (read more)

Annotated medical data is a prerequisite for successful and robust deep-learning models. However, the curation of labels for medical images is... (read more)
Surgery & Intervention 4.0

Death within 30 days after surgery has recently been found to be the third-leading cause of death worldwide [1], with research suggesting... (read more)
Models for Personalized Medicine

Pharmacogenomics (PGx) studies how variations in the genome affect drug response in patients. There are genomic variants in so-called pharmacogenes which... (read more)

In order to build machine learning models for predictive healthcare, the standard approach is to learn a unified prediction model on... (read more)
Projects 2021
Imaging & Diagnostics

During the ongoing COVID-19 pandemic, probabilistic forecasts of infectious disease spread have become a research priority, because they allow for a... (read more)

During the last decade, single-cell omics technologies such as single-cell transcriptomics (SCT) have rapidly emerged. As a complement to cultivation-based meta-transcriptomic... (read more)
Surgery & Intervention 4.0
Half of all cancer patients receive radiation therapy which delivers high-energetic, ionizing radiation to target cancerous tissue while sparing healthy tissue... (read more)

Machine Learning (ML) models are increasingly diffusing in healthcare. ML models can, for example, come into play in cognitive surgical robots... (read more)
Models for Personalized Medicine
The goal is to develop machine learning strategies for deciphering cell-of-origin related cancer heterogeneity. Building on variational autoencoders and related dimensionality... (read more)

Collagen is the most abundant protein in our body and performs a variety of functions, including strengthening and supporting skin, tendons... (read more)

Protein-biomolecule-interactions are ubiquitous in life, from DNA-replication to regulating the heartbeat. The stability of biomolecular complexes depend heavily on the involved... (read more)
Projects 2020
Imaging & Diagnostics

Cell segmentation and tracking is the problem of processing a time series of (3D) images showing development of an organism (e.g... (read more)

Tumour hypoxia, a state of low oxygen levels in certain tissue regions, seems to play a prognostic role for loco-regional tumour... (read more)

Since reliable biomarkers for psychiatric diagnosis and treatment indication are lacking, such diagnostics and prognostics is mainly based on structural interviews... (read more)
Surgery & Intervention 4.0

The precise spatial delineation of cancerous and healthy tissue in radiation therapy is necessary to prevent side effects and the reoccurrence... (read more)

Laparoscopic surgery is a team effort. A surgeon and her assistant(s) collaborate to solve a shared task working individually and as... (read more)

Death within 30 days after surgery has recently been found to be the third-leading cause of death worldwide. In this context... (read more)
Models for Personalized Medicine

As it is becoming progressively challenging to wholly analyse the ever-increasing amounts of generated biomedical data (e.g., CT scans, X-ray images... (read more)

In recent years, biomedical data have been increasingly available. In particular, the costs for procuring omics data, including genome and exome... (read more)

A deep understanding of tissue growth as emerging behavior from single-cell events might lead to new insights for different scientific fields... (read more)

The accurate diagnosis of patients with rare diseases is important but challenging, since the prevalence of them is very low. This... (read more)
Projects 2019
Imaging & Diagnostics

While Deep Learning approaches, in particular Convolutional Neural Networks (CNNs), are now also being widely adopted in medical image analysis, only... (read more)
Death within 30 days after surgery has recently been found to be the third-leading cause of death worldwide. One of the... (read more)

Fluorescence microscopy has several inherent limitations, which are dictated by basic optical and physical laws as well as compromises arising from... (read more)
Many biomedical imaging devices are able to collect time series of high resolution 3D data (3D+t). A typical task in these... (read more)

Compared to man-made technical control systems, biological control systems exhibit a remarkable fault tolerance over wide ranges of environmental cues as... (read more)

The image shows the challenge of the project in my view: the first part shows a volume rendering of a lung... (read more)

An assumption-free automatic check of medical images for potentially overseen anomalies would be a valuable assistance for a radiologist. Deep learning... (read more)
Surgery & Intervention 4.0
Machine learning-based decision support can potentially improve the quality of healthcare by providing physicians with the right information at the right... (read more)

Radiation therapy is one of the cornerstones in modern cancer treatment being applied in 50 % of all patients. It is a... (read more)
Current Machine Learning algorithms, especially Deep Neural Networks (DNNs), have shown to be successful tools in areas particularly relevant for Life... (read more)

Photoacoustic imaging (PAI) is an emerging modality that has the potential to provide tomographic images of blood oxygenation - an important... (read more)
Models for Personalized Medicine

Modern life sciences with their highly sensitive omics data sets face several challenges with regard to data storage and sharing. On... (read more)

With the possibility of whole genomic sequencing for oncologic patients, many processes in their treatment have to be adapted. Physicians in... (read more)
Multi-omics, the generation of omics-profiles with multiple assays on the same set of biological samples, is a fundamental experimental design pattern... (read more)

Publications

Steering Committee
Spokespersons

Coordination Office
Coordination

Data Science Groups

Till Bärnighausen is Alexander von Humboldt Professor and Director of the Heidelberg Institute of Global Health (HIGH), Heidelberg University, Germany. He is also Senior Faculty at the Africa Health Research Institute (AHRI) in South Africa and fellow at the Harvard Center for Population and Development Studies. Till's research focuses on creating and evaluating global health interventions. His research has been funded by the US National Institutes of Health, European Union, German Research Foundation, Alexander von Humboldt Foundation, Wellcome, Bill & Melinda Gates Foundation, Clinton Health Access Initiative, Else-Kröner-Fresenius Foundation, USAID, UNAIDS, WHO, KfW, World Bank. Till has previously worked as professor at Harvard T.H. Chan School of Public Health; as medical doctor in Germany, China and South Africa; and as management consultant for McKinsey & Company. Till holds doctoral degrees in international and population health (Harvard) and history of medicine (Heidelberg), and master degrees in health systems management (LSHTM), financial economics (SOAS), and innovation and entrepreneurship (HEC Paris).

Life Science Groups

Our aim is to obtain genomes from new bacterial and archaeal taxa with no sequenced representatives and providing direct link information between cell's phylogenetic and metabolic markers by matching phylogeny and function. We unravel novel metabolisms, ecophysiology and mechanisms of energy conservation among uncultured microorganisms and in addition investigating minimal genome requirements and syntrophic interactions as well as genetic and phenotypic heterogeneity among cells, cell-to-cell variations, horizontal gene transfer and evolutionary pressure.

The Durstewitz group develops statistical machine learning methods at the mathematical and algorithmic levels, with a focus on nonlinear time series analysis, nonlinear dynamical systems, and generative recurrent neural networks. Our main application domains are functional neuroimaging and electrophysiological data, as well as smartphone-based ecological momentary assessments, in psychiatry and neurology.

Our research focuses on (a) pattern recognition in biological data, multi-omics analysis and data integration with special application to cancer genomics as well as (b) translational and personalized oncology, molecular tumor boards, biomarker development and data analysis for clinical trials.

Our research focuses on using data science for studying drivers and predicting the emergence of infectious disease spreading among animals, insects, and humans. Specifically, we use and develop process-based modelling, machine learning and combination approaches integrating data sources from different disciplines and domains across biological, environment, and social domains to explain, forecast and project risks. Within this area we are engaged in larger international networks and projects on infectious disease ecology and dynamics, pandemic preparedness and climate change impacts and adaptation.

We develop methods and tools enabling data-driven insights into cancer biology through the epigenomes of tumor cells. Our major research topics include integrative analysis of large epigenomic data sets, deconvolution approaches for tumour heterogeneity inference, novel experimental techniques for epigenome profiling, as well as generic infrastructure for reproducible bioinformatics.

Our main aim is to develop and apply computer-aided methods to study how biomolecules, such as proteins, interact. The methods make use of three-dimensional macromolecular structures and combine multiscale approaches based on physicochemical principles with those of bio-/chemo-informatics and machine learning.

We are interested in inborn errors of metabolism with a special focus on defects in neurotransmitter biosynthesis and metabolism including defects in the biosynthesis of dopamine, serotonin, and GABA, as well as in tetrahydrobiopterin (BH4) biosynthesis. We use patient-specific induced pluripotent stem cells (iPSCs), CRISPR-generated isogenic control iPSC lines and differentiated somatic cell types (neurons, glia) and organoids to elucidate the pathophysiology of the diseases. We are especially interested in (a) gaining a deeper understanding of the complex pathophysiology of the diseases, (b) obtaining better genotype/phenotype correlations and (c) the identification of new therapeutic targets and diagnostic markers.

Training Summary
Overview
The goal of the HIDSS4Health curriculum is that its doctoral researchers become experts in data science and in at least one health-related field. It will include the ability to communicate with experts from both sides and to transfer knowledge from data to life science and vice versa. All lectures, courses, retreats and summer schools are held in English.
Supervision Concept
All doctoral researchers will receive feedback from a Thesis Advisory Committee (TAC). This consists of an interdisciplinary team of at least three Principal Investigators (PI), including PIs with data science and life science backgrounds from different institutions. TAC meetings will discuss the progress of the doctoral research project, the publication concept, working plans, cooperation potential with other doctoral researchers, planning of the mobility phase, as well as the supervision quality and career perspectives of the doctoral researcher. Although each doctoral researcher will belong to the main supervisor’s group, we recommend an associate membership in the group of a second PI, including an official second affiliation, regular stays in this group (such as once a week or one week per month) and integration in lab meetings etc. We anticipate that this will foster closer integration between data and life science expertise and a deeper understanding of both aspects.
Degree
In general, the doctoral thesis degree will be awarded by the informatics faculty of KIT or Heidelberg University, depending on your main supervisor’s individual affiliation. Each doctoral researcher who successfully finishes all training modules and the doctoral thesis project will receive a certificate as “Data Scientist”, including a confirmation regarding specialization in the health-related application domain. This certificate is a joint activity of the Faculty for Informatics at KIT and the Faculty for Mathematics and Computer Science at Heidelberg University.
Lectures, Workshops, Seminars
All incoming doctoral researchers start with the interdisciplinary course “Data Science & Health”. This is held by the PIs of the school and invited speakers who cover additional topics. The course consists of lecture-style elements and an interactive discussion and covers data science methods (such as clustering, image analysis, visualization, deep learning), domain-oriented methods (such as medical imaging, surgery) as well as ethical, legal and social implications. In addition, it will train researchers to consider the different views and needs of data science and health-related sciences, and the opportunities for interdisciplinary cooperation.
The following lecture course "Advanced Topics in Data Science & Health" has two objectives. On the one hand, it provides advanced doctoral researchers and postdocs with the opportunity to gain some experience in this type of teaching format. On the other hand, it will provide first-year doctoral researchers with an overview of recent scientific developments and the state of the art in the research fields of the presenters.
In addition, we will offer a set of specific scientific courses (for example, on Simulation & Optimization, Machine Learning, Uncertainty Quantification, Scientific Visualization, Python, Confocal Microscopy, International Zebrafish and Medaka Course) with a data and/or life science orientation.
The exact configuration of these compulsory elective courses is individually chosen by the doctoral researcher depending on thematic needs and existing skills.
Exchange Program
Each doctoral researcher of the school should complete a funded mobility phase of around three months, either at a leading international university or institution. The school supports the selection of appropriate partner institutions, for example, using existing international cooperation networks such as HeKKSaGOn (the Heidelberg – Kyoto – Karlsruhe – Sendai – Göttingen – Osaka network of German and Japanese universities) and InterACT (network of eight universities from Europe, Asia and America including KIT and Carnegie Mellon University). DKFZ has exchange programs with the Weizmann Institute of Science (Israel), the MD Anderson Cancer Center (USA) and partners of Cancer Core Europe.
Summer Schools
Each doctoral researcher is expected to attend at least one summer school, which will be held annually. These summer schools will be co-organized with other data science schools within HIDA, thus enabling all Helmholtz Information and Data Science School participants to network and encourage further collaboration.
Personal Skill Training
The school provides a wide portfolio of personal skills training (including leadership and language) as well as comprehensive support measures regarding internationalization, networking and career orientation. Courses such as “Introduction to German Academic Culture”, “Publishing in Scientific Journals”, and “Managing Projects” are currently offered. Furthermore, research school doctoral researchers will be encouraged to participate in the centrally-organized Helmholtz Transferable Skills Training program for doctoral researchers. Three modules, each lasting 2–3 days will provide training in (I) research skills development, (II) presentation and communication and (III) career and leadership.
Cross Theme Topics
Cross Theme Topics (CTTs) are a collaboration between 2–4 doctoral researchers working on related methodological problems, supported and mentored by postdocs and PIs of the school. The aim of a CTT is to leverage synergy potentials between projects (for example by sharing special skills of a doctoral researcher between projects) and to broaden the individual methodological understanding beyond the research areas. The ideal outcome of a CTT would be a joint paper, software tool or benchmark dataset. All doctoral researchers in HIDSS4Health will be encouraged to be involved in at least one CTT during his/her time in the school.

Training Program
Lectures
Data Science & Health – Winter Semester 2023/2024
⎙This lecture is held by the PIs of the school and invited speakers who cover additional themes. The course consists of lecture-style elements and an interactive discussion. It covers data science methods (e.g., clustering, image analysis, visualization, deep learning), domain-oriented methods (e.g., medical imaging, surgery) as well as ethical, legal and social implications. The aim of the course is to introduce the doctoral researchers to the field and to give a basic understanding of all research areas of the school. In addition, it will train them to consider the different views and needs of data science and health-related sciences, and the opportunities for interdisciplinary cooperation.
Organization
Dates ⓘ
Date | Time | Location | Lecturer | Topic |
---|---|---|---|---|
2023-10-26 | 14:00–15:30 | Zoom | Pascal Friederich | Bayesian Optimization for Autonomous Experiments |
2023-11-02 | 14:00–15:30 | Zoom | Emilia Graß | Cybersecurity in Healthcare |
2023-11-09 | 14:00–15:30 | Zoom | Alexander Schug | Inference on Biomolecular Data by Machine Learning |
2023-11-16 | 14:00–15:30 | Zoom | Ralf Mikut | Time Series Analysis |
2023-11-23 | 14:00–15:30 | Zoom | Vincent Heuveline | Uncertainty Quantification (UQ) for medical models |
2023-11-30 | 14:00–15:30 | Zoom | Junyan Lu | Multi-omics data integration for precision medicine |
2023-12-07 | 14:00–15:30 | Zoom | Martin Frank | Mathematical Foundations of Deep Learning |
2023-12-14 | 14:00–15:30 | Zoom | Stefan Riezler | Reproducibility of Machine Learning Research |
2023-12-21 | 14:00–15:30 | Zoom | Rainer Stiefelhagen | TBD |
2024-01-11 | 14:00–15:30 | Zoom | Julio saez-Rodriguez | Knowledge-based machine learning from omics data |
2024-01-18 | 14:00–15:30 | Zoom | Joacim Rocklöv | Integrated Surveillance and Predictions for Prepardness |
2024-01-25 | 14:00–15:30 | Zoom | Johannes Bracher / Melanie Schienle | Evaluation of probabilistic forecasts in infectious disease modelling |
2024-02-01 | 14:00–15:30 | Zoom | Anne Kaster | Single Cell Omics. |
2024-02-08 | 14:00–15:30 | Zoom | Shiva Faeghi | Process Mining in Healthcare |
2024-02-15 | 14:00–15:30 | Zoom | Evangelia Christodoulou / Annika Reinke | Validation Matters: Pitfalls and recommendations for model evaluation in Biomedical Imaging Analysis |
Six Main Tasks in Image Processing – Summer Semester 2023
⎙In this series of seminars, six key image processing tasks will be discussed, following a typical workflow in the image processing pipeline. Images are not always captured by a camera. Often, they must be tediously reconstructed from a series of projections or other non-image types of acquisitions. Different reconstruction algorithms allow for better image quality or can focus on specific properties of the objects under observation. Noise can be introduced at many steps in the image acquisition process. Denoising is therefore an essential step in most image processing workflows. Tracking individual objects over multiple time steps is a difficult task, but allows for the observation of temporal dynamics. Segmentation refers to the assignment of each pixel in an image to a specific category. In semantic segmentation, all pixels belonging to a cat are labeled "cat", and all pixels belonging to trees are labeled "tree". In instance segmentation, each pixel is additionally assigned to an object instance, making it possible to distinguish multiple cats and trees in an image. The visualization of otherwise difficult-to-interpret data, such as reconstructed 3D(+T) objects or high-dimensional image data, is essential for understanding the results. Finally, interpreting the results of AI-based image analysis algorithms is important: Why was a particular decision made? What structures in the images were responsible? What can AI tell us about the underlying problem?
This lecture series is held by imaging experts invited by the organizing schools and Helmholtz Imaging. The course consists of presentations and interactive discussions. It covers different imaging techniques in life sciences and soft matter. For an in-depth understanding of the subject, we recommend attending all lectures. Registration is mandatory for participation. Places are limited and in the case of overbooking, priority will be given to fellows (members) of the three schools. Participants demonstrating an attendance record of more than 70% can receive a certificate of participation.
The lecture series is offered online. It takes place (more or less) bi-weekly. Registration is required; a registration link can be requested via mail to sabine.niebel@helmholtz-imaging.de.
Further details and up-to-date information in case of changes: https://events.hifis.net/event/793/
Date | Time | Location | Lecturer | Topic |
---|---|---|---|---|
2023-05-04 | 14:00–15:30 | Zoom | Prof. Philip Kollmannsberger (HHU) | Six Main Tasks in Image Processing: an Overview |
2023-05-25 | 14:00–14:45 | Zoom | Dr. Christoph Lerche (FZJ) | Tomographic Methods in Medical Imaging |
2023-05-25 | 14:45–15:30 | Zoom | Dr. Tim-Oliver Buchholz (FMI) | Restoring Noisy Microscopy Images |
2023-06-15 | 14:00–15:30 | Zoom | Prof. Carsten Rother (HCI) | Tracking of Objects: from One to Many |
2023-06-29 | 14:00–15:30 | Zoom | Prof. Dagmar Kainmüller (MDC) | Machine Learning for Image Segmentation |
2023-07-06 | 14:00–15:30 | Zoom | Deborah Schmidt (MDC) | Visualization |
2023-07-27 | 14:00–15:30 | Zoom | Prof. Ullrich Köthe (IWR) | Explainable Machine Learning |
Advanced Topics in Data Science & Health – Summer Semester 2023
⎙This lecture is held by doctoral researchers of HIDSS4Health in their second or third year. The course if offered online and mainly for the HIDSS4Health doctoral researchers, but other doctoral researchers interested in single topics can request a registration link via mail to office@hidss4health.de.
Date | Time | Location | Lecturer | Topic |
---|---|---|---|---|
2023-04-25 | 14:00–14:45 | Zoom | Tim Ortkamp | Bayesian Hyperparameter Optimization |
2023-04-25 | 14:45–15:30 | Zoom | Simon Warsinsky | Improving Data Quality in Medical Image Annotation Tasks through Gamification |
2023-05-02 | 14:00–14:45 | Zoom | Abdul Moeed | Causality and Machine Learning |
2023-05-02 | 14:45–15:30 | Zoom | Balazs Gyenes | Model-based Reinforcement Learning |
2023-05-09 | 14:00–14:45 | Zoom | Robin Fleige | Multi-Parameter Bifurcation Analysis of Vector Fields |
2023-05-09 | 14:45–15:30 | Zoom | Julia Münch | Next Generation Sequencing - Application and Data Analysis |
2023-05-16 | 14:00–14:45 | Zoom | Yannick Kirchhoff | Self-configuring methods for biomedical image analysis tasks |
2023-05-16 | 14:45–15:30 | Zoom | Marlen Neubert | Machine Learning for Molecular Simulations |
2023-05-23 | 14:00–14:45 | Zoom | Daniel Wolffram | Probabilistic Forecasting |
2023-05-23 | 14:45–15:30 | Zoom | Sebastian Pirmann | Cancer Pharmacogenomics |
2023-05-30 | 14:00–14:45 | Zoom | Reiner Dolp | TBA |
2023-05-30 | 14:00–14:45 | Zoom | Max Piochowiak | Volume Visualization |
2023-06-06 | 14:00–14:45 | Zoom | Marcel Meyer | Boltzmann Generators |
2023-06-06 | 14:00–14:45 | Zoom | Ahmad Bin Qasim | TBA |
2023-06-13 | 14:00–14:45 | Zoom | Jayson Salazar Rodriguez | TBA |
Data Science & Health – Winter Semester 2022/2023
⎙This lecture is held by the PIs of the school and invited speakers who cover additional themes. The course consists of lecture-style elements and an interactive discussion. It covers data science methods (e.g., clustering, image analysis, visualization, deep learning), domain-oriented methods (e.g., medical imaging, surgery) as well as ethical, legal and social implications. The aim of the course is to introduce the doctoral researchers to the field and to give a basic understanding of all research areas of the school. In addition, it will train them to consider the different views and needs of data science and health-related sciences, and the opportunities for interdisciplinary cooperation.
Organization
Dates ⓘ
Date | Time | Location | Lecturer | Topic |
---|---|---|---|---|
2022-10-25 | 14:00–15:30 | Zoom | Leonardo Ayala | Optical imaging concepts enabled by deep learning |
2022-11-08 | 14:00–15:30 | Zoom | Junyan Lu | Multi-omics data integration for precision medicine |
2022-11-15 | 14:00–15:30 | Zoom | Alexander Schug | Data inference in molecular biology: from mutual information to alpha fold |
| ||||
| ||||
2022-12-06 | 14:00–15:30 | Zoom | Pascal Friederich | Bayesian Optimization for Autonomous Experiments |
2022-12-13 | 14:00–15:30 | Zoom | Ullrich Köthe | Bayesian Inference with invertible neural networks |
2022-12-20 | 14:00–15:30 | Zoom | Mark Ladd | Imaging Physics in Oncology |
2023-01-10 | 14:00–15:30 | Zoom | Ali Sunyaev | Distributed Ledger Technology in genomics |
2023-01-17 | 14:00–15:30 | Zoom | Martin Frank | Mathematical Foundations of Deep Learning |
2023-01-24 | 14:00–15:30 | Zoom | Michael Gertz | Data Science for Text Analysis |
2023-01-31 | 14:00–15:30 | Zoom | Shiva Faeghi | Process Mining in Healthcare |
2023-02-07 | 14:00–15:30 | Zoom | Ralf Mikut | Time Series Analysis |
2023-02-14 | 14:00–15:30 | Zoom | Emilia Grass | Cybergeddon in Healthcare: Preparing for the Worst |
Note
Imaging – From Organisms to Molecules – Summer Semester 2022
⎙(Lecture Series on Imaging Methods and Applications in Life Sciences and Soft Matter)
The application areas of imaging in life sciences and soft matter are almost inexhaustible in number. Highly specialized techniques and methods have thus been developed over time for imaging and analyzing a wide variety of samples, giving us unique insights into their structures at all scales. Over the course of this lecture series, data scientists and domain scientists will introduce you to a great number of imaging techniques.
Organization
Dates ⓘ
Date | Time | Location | Lecturer | Topic |
---|---|---|---|---|
2022-04-28 | 14:00–15:30 | Online | Mark Ladd | Imaging Physics in Medicine |
2022-05-12 | 14:00–15:30 | Online | Paul Jäger | Analyzing Medical Images Using Machine Learning |
2022-06-02 | 14:00–15:30 | Online | Michael Wagner | Optical Coherence Tomography in Biofilm Research – Visualization and Characterization of the Mesoscopic Biofilm Structure |
2022-06-23 | 14:00–15:30 | Online | Rudolf Merkel | Basics of Light Microscopy for the Study of Cells |
2022-06-30 | 14:00–15:30 | Online | Gerd Ulrich Nienhaus | Advanced Fluorescence Microscopy |
2022-07-07 | 14:00–15:30 | Online | Lennart Hilbert | Microscopy Assessment of DNA-based Information Processing in Biological and Artificial Systems |
2022-07-14 | 14:00–15:30 | Online | Carsten Sachse | Imaging Biological Molecules by Electron Cryo-Microscopy (cryo-EM) |
Advanced Topics in Data Science & Health – Summer Semester 2022
⎙This lecture is held by doctoral researchers of HIDSS4Health in their second year. The course if offered online and mainly for the HIDSS4Health doctoral researchers, but other doctoral researchers interested in single topics can request a registration link via mail to office@hidss4health.de.
Date | Time | Location | Lecturer | Topic |
---|---|---|---|---|
2022-04-27 | 16:00–16:45 | Zoom | Verena Bitto | Statistics done wrong |
| ||||
2022-05-04 | 16:00–16:45 | Zoom | Stefan Haller | Lagrange Decomposition for Combinatorial Optimization Problems |
2022-05-04 | 16:45–17:30 | Zoom | Alexandra Walter | Optimization of Artificial Neural Networks: Mathematics behind Stochastic Gradient Descent |
2022-05-11 | 16:00–16:45 | Zoom | Elaine Zaunseder | The needle in the haystack - how to handle imbalanced medical data |
2022-05-11 | 16:45–17:30 | Zoom | Philipp Toussaint | Explainable AI - Methods for Interpretability and Explainability in ML and DL |
2022-05-18 | 16:00–16:45 | Zoom | Philipp Wimmer | Visualization of Scientific Data with Paraview |
2022-05-18 | 16:45–17:30 | Zoom | Paul Maria Scheikl | Reinforcement Learning for Surgical Robotics |
2022-05-25 | 16:00–16:45 | Zoom | Vahdaneh Kiani | Introduction to medical image registration |
2022-05-25 | 16:45–17:30 | Zoom | Alejandra Jayme | Hypernetworks for CNN or Bayesian Neural Networks |
2022-06-01 | 16:00–16:45 | Zoom | Julian Herold | Is Attention All You Need? (Intro to Transformers) |
Data Science & Health – Winter Semester 2021/2022
⎙This lecture is held by the PIs of the school and invited speakers who cover additional themes. The course consists of lecture-style elements and an interactive discussion. It covers data science methods (e.g., clustering, image analysis, visualization, deep learning), domain-oriented methods (e.g., medical imaging, surgery) as well as ethical, legal and social implications. The aim of the course is to introduce the doctoral researchers to the field and to give a basic understanding of all research areas of the school. In addition, it will train them to consider the different views and needs of data science and health-related sciences, and the opportunities for interdisciplinary cooperation.
Organization
Dates ⓘ
Date | Time | Location | Lecturer | Topic |
---|---|---|---|---|
2021-10-19 | 14:00–15:30 | Zoom | Klaus Maier-Hein | Medical Image Computing |
2021-10-26 | 14:00–15:30 | Zoom | Ralf Mikut | Time Series Analysis |
2021-11-02 | 14:00–15:30 | Zoom | Alexander Schug | Data Inference on Sequence Data: How to predict biomolecular structure and function |
2021-11-09 | 14:00–15:30 | Zoom | Michael Beigl | Wearable Health Data |
2021-11-16 | 14:00–15:30 | Zoom | Mark Ladd | Imaging Physics in Oncology |
2021-11-23 | 14:00–15:30 | Zoom | Pascal Friederich | Graph Neural Networks for Molecular Design |
2021-11-30 | 14:00–15:30 | Zoom | Anne Kaster | Single Cell Omics |
2021-12-07 | 14:00–15:30 | Zoom | Lennart Hilbert | Fluorescence microscopy and digital image processing in molecular cell biology |
2021-12-14 | 14:00–15:30 | Zoom | Franziska Mathis-Ullrich | Co-Operation with Surgical Robots |
2021-12-21 | 14:00–15:30 | Zoom | Oliver Jäkel | Why data sciences will be crucial for modern image guided radiotherapy |
2022-01-11 | 14:00–15:30 | Zoom | Annika Reinke | Next-generation biomedical image analysis competitions |
2022-01-18 | 14:00–15:30 | Zoom | Michael Gertz | Trends and Topics in Text Analytics |
| ||||
2022-02-01 | 14:00–15:30 | Zoom | Oliver Stegle | Machine learning for genomics |
2022-02-08 | 14:00–15:30 | Zoom | Carsten Dachsbacher | Introduction to Visual Data Science |
Data Science & Health – Winter Semester 2020/2021
⎙This lecture is held by the PIs of the school and invited speakers who cover additional themes. The course consists of lecture-style elements and an interactive discussion. It covers data science methods (e.g., clustering, image analysis, visualization, deep learning), domain-oriented methods (e.g., medical imaging, surgery) as well as ethical, legal and social implications. The aim of the course is to introduce the doctoral researchers to the field and to give a basic understanding of all research areas of the school. In addition, it will train them to consider the different views and needs of data science and health-related sciences, and the opportunities for interdisciplinary cooperation.
Organization
Data Science & Healthis offered online. The lecture takes place every two weeks and only attendance is documented. Currently, no further examinations are planned. Registration is required, a registration link can be requested via mail to office@hidss4health.de.
Dates ⓘ
Date | Time | Location | Lecturer | Topic |
---|---|---|---|---|
2020-11-02 | 14:00–15:30 | Zoom | Bogdan Savchynskyy | Combinatorial Optimization Techniques for Bioimaging |
2020-11-02 | 15:45–17:15 | Zoom | Frank Ückert | Medical Informatics in Translational Oncology |
2020-11-16 | 14:00–15:30 | Zoom | Ralf Mikut | Time Series Analysis |
2020-11-16 | 15:45–17:15 | Zoom | Alexander Schug | Data Inference on Sequence Data |
2020-11-30 | 14:00–15:30 | Zoom | Holger Fröning | Any Growth is Bounded – On the Future of Performance Scaling |
2020-11-30 | 15:45–17:15 | Zoom | Benedikt Brors | The n<<p paradigm in omics data analysis |
2020-12-14 | 14:00–15:30 | Zoom | Franziska Mathis-Ullrich | Minimally-Invasive Robots for Medicine |
2020-12-14 | 15:45–17:15 | Zoom | Martin Frank | Mathematical Foundations of Deep Learning |
2021-01-11 | 14:00–15:30 | Zoom | Michael Beigl | Wearable and mobile health data |
2021-01-11 | 15:45–17:15 | Zoom | Filip Sadlo | Introduction to Visual Data Science |
2021-01-25 | 14:00–15:30 | Zoom | Peter Sanders | Parallel Algorithms for Dummies |
2021-01-25 | 15:45–17:15 | Zoom | Achim Streit | Introduction to Distributed and Parallel Computing |
2021-02-08 | 14:00–15:30 | Zoom | Lena Maier-Hein | Surgical Data Science |
2021-02-08 | 15:45–17:15 | Zoom | Michael Gertz | Trends and Topics in Text Analytics |
Data Science & Health – Winter Semester 2019/2020
⎙This lecture is held by the PIs of the school and invited speakers who cover additional themes. The course consists of lecture-style elements and an interactive discussion. It covers data science methods (e.g., clustering, image analysis, visualization, deep learning), domain-oriented methods (e.g., medical imaging, surgery) as well as ethical, legal and social implications. The aim of the course is to introduce the doctoral researchers to the field and to give a basic understanding of all research areas of the school. In addition, it will train them to consider the different views and needs of data science and health-related sciences, and the opportunities for interdisciplinary cooperation.
Organization
The lectures take place at the following locations:
- KIT: Geb 30.28, seminar room 004, Wolfgang-Gaede-Straße 6, 76131 Karlsruhe
- Mathematikon: Interdisziplinäres Zentrum für Wissenschaftliches Rechnen IWR, conference room 5.104, Im Neuenheimer Feld 205, 69120 Heidelberg
- DKFZ: main building, room H824 (8th floor), Im Neuenheimer Feld 280, 69120 Heidelberg
Dates ⓘ
Date | Time | Location | Lecturer | Topic |
---|---|---|---|---|
2019-10-22 | 09:45–11:15 | KIT | Ralf Mikut | Time Series Analysis |
2019-10-22 | 11:30–13:00 | KIT | Lennart Hilbert | Fluorescence microscopy and digital image processing in molecular cell biology |
2019-11-05 | 09:45–11:15 | Mathematikon | Carsten Rother | Image-based Machine Learning |
2019-11-05 | 11:30–13:00 | Mathematikon | Klaus Maier-Hein | Radiologic Data Science |
2019-11-19 | 09:45–11:15 | KIT | Achim Streit | Introduction to Distributed and Parallel Computing |
2019-11-19 | 11:30–13:00 | KIT | Peter Sanders | Parallel Algorithms for Dummies |
2019-12-03 | 09:45–11:15 | Mathematikon | Holger Fröning | Any Growth is Bounded – On the Future of Performance Scaling |
2019-12-03 | 11:30–13:00 | Mathematikon | Lena Maier-Hein | Surgical Data Science |
2019-12-17 | 09:45–11:15 | KIT | Franziska Mathis-Ullrich | Minimally-Invasive Robots for Medicine |
2019-12-17 | 11:30–13:00 | KIT | Tamim Asfour | Data-Driven Learning of Sensorimotor Skills for Robots and Exoskeletons |
2020-01-07 | 09:45–11:15 | Mathematikon | Michael Gertz | Introduction to Text Analysis |
2020-01-07 | 11:30–13:00 | Mathematikon | Robert Strzodka | Essential Performance Considerations in Programming |
2020-01-21 | 09:45–11:15 | KIT | Anne Koziolek | Requirements Engineering for Data-driven Solutions |
2020-01-21 | 11:30–13:00 | KIT | Ali Sunyaev | Introduction to Decentralized Data Management with Distributed Ledger Technology |
2020-02-04 | 09:45–11:15 | DKFZ | Martin Frank | Mathematical Aspects of Uncertainty Quantification |
2020-02-04 | 11:30–13:00 | DKFZ | Filip Sadlo | Introduction to Visual Data Science |
Seminars
Scientific Writing Course
Workshop Content- Five stages of Publishing
- Who is my reader?
- Destination and Roadmap
- Building structure and connectivity
- From scientific report to a scientific argument
- Scientific writing in English – words, sentences, and paragraphs
Training Methods
This is a highly interactive online training workshop with extensive elements of partner work, exercises, group discussion, and including some offline homework tasks. We use innovative online tools combined with proven didactic techniques to reproduce as much as possible a real workshop situation. We place a special emphasis on sharing and learning from participants’ own expertise and experience. To increase impact and applicability, we work with real-life cases from the participants whenever possible. We may ask participants to reserve time for individual preparatory work before the workshop, and between each session.
Workshop Goal
The goal of this workshop is to help publishing scientists develop a more impartial,analytical view of scientific writing, to better understand their readers as the focus for their scientific communication, and to make them more efficient writers and editors. Their writing will no longer be driven by a standard formula for How? to write a paper, but will be inspired by the question Why? Participants will develop a deeper understanding of the structure of scientific papers, with a renewed focus on the purpose of each section and the connections between them. They will gain a global framework for conceptualising the entire publishing process, how to create an expectation in the reader and then deliver on that expectation, and how to make the qualitative jump from a passive scientific account to an active scientific argument. Finally, we will explore some common problems of language construction that make scientists’ writing unclear, and why we are prone to these problems; we will practice some intuitive editing tools to address them.
This course will take place online on 06. – 08. February 2023 from 9:30-13:00 each day. A total of 16 people can participate. If you would like to participate, please contact (office@hidss4health.de) by 08. November 2022 at the latest.
Managing a Constructive Relationship between PhD Students and their Supervisors
You are currently doing your doctorate and it often seems difficult to manage the relationship between yourself and your supervisor? You need support and/or feedback, but it’s hard to get an appointment at all? You feel left alone with your doctoral thesis? You work very hard and sometimes even feel drained? It’s time to clarify the relationship and to find a satisfactory way to manage the expectations between both parties, PhD students and their supervisors.
The workshop takes place online on March 3rd 2022, from 9 a.m. to 5 p.m. and includes a lot of practical sessions.
For registration, please visit the course website.

Apply
Overall Process
The HIDSS4Health research school performs an annual candidate selection process. Suitable candidates are selected in a three-step process: (1) the written application, (2) a virtual one-day selection event for short-listed applicants, and (3) matching projects and candidates. Evaluation criteria for the candidates are an excellent academic record and a strong motivation for the interdisciplinary nature of the projects. The institutions are committed to increasing the percentage of female scientists and encouraging female applicants to apply. The next application call is anticipated for December 2023/January 2024.
Requirements
We are looking for excellent graduates holding master degrees in computer science, mathematics, engineering, physics or related quantitative sciences (e.g., bioinformatics or medical informatics).
As an international research school, we require our doctoral researchers to be fluent in English (German is optional). If you are neither a native German nor a native English speaker, we therefore ask for a proof of language skills in form of a certificate (IELTS, TOEFL or CAE) or a certified statement that the studies in your previous university degree were taught in English.
Funding
All positions are fully-funded (e.g. “E13” TVöD or TV-L positions under applicable regulations of the participating institutions) according to the public sector salary system at German universities and research centers. It means a yearly gross income of more than 45.000 € and results in more than 26.000 € net income after taxes, own contributions to health insurance, pension insurance, unemployment insurance etc. Weekly working times are 39.5 hrs, the contract includes 30 paid leave days. However, since the German system is quite complicated, all financial details cannot be provided here but can be calculated here (sorry, only available in German).
Living in Heidelberg / Karlsruhe
Heidelberg and Karlsruhe are attractive cities located in the sunny south-western part of Germany within a traveling distance of around 50 kilometers. They are connected via a dense public transport network. More information on living costs can be found in the information pages of the German Academic Exchange Service (DAAD) or in a PDF booklet from the German Federal Ministry for Education and Research.
Diversity and Equal Opportunities
The provision of equal opportunities and diversity is a central concern of KIT, DKFZ and Heidelberg University. We are committed to enabling researchers to balance the demands of career and family life and therefore offer various options to staff, such as flexible working time models, family-friendly meeting times, re-entry after maternity leave, comprehensive childcare concepts, provision of Tele-offices, parent-child offices, and in-house childcare facilities. Funded places in local childcare facilities offer full-time and part-time child-care (for example during a conference or summer school). The networks of female scientists at the partners – “WiKIT” at the KIT and the Executive Women’s Initiative at the DKFZ – are professional networks of female scientists in leading positions. These networks are platforms of mutual exchange and aim at fostering networking among female scientists of various disciplines to improve the career perspectives and working conditions of female scientists in Germany. All measures promoting the compatibility of career and family life are monitored and advanced by the “familiengerechte Hochschule” (family-friendly university) audit and “Audit Beruf und Familie” (Career and Family Audit) at the KIT, Heidelberg University and DKFZ, respectively.
Becoming an Associated Doctoral Researcher
You are already funded and your doctoral research is on a topic that is relevant to the school? Apply for an association!
Supporting Early Carreer Female Scientist
Do you have interesting projects and want to supervise a PhD in the context of HIDSS4Health? Then apply now as we are looking for early career female scientists who want to become PIs eligible to apply for projects in HIDSS4Health (deadline October 2021).

Events
Upcoming Events
Helmholtz GPU Hackathon
- 10 days
- Online
- Website
HIDSS4Health Selection Event
- 1 day
- Online
Third round internal selection event for invited candidates (online format).
Visualizing Science
- 2 days
- Online
- Website
Presentation Skills Course
- 3 days
- Online
- Website
HIDSS4Health Retreat
- 3 days
- Bad Herrenalb
Retreat for doctoral researchers of the school.
Helmholtz Data Science Career Day
- 1 day
- Online
- Website
Doctoral Researcher General Assembly
- 1 day
- DKFZ
General assembly event for the doctoral researchers of the school, 13:00–17:00 (internal event).
Workshop Time and Self-Management
- 1 days
- Heidelberg
- Website
HIDSS4Health General Assembly
- 1 day
- Online
General assembly event of the school, 09:00–12:00 (internal event).
Workshop Time and Self-Management
- 1 days
- Online
- Website
DASHH Data Science Colloquium
- 1 days
- Online
- Website
Introduction to machine learning
- 3 days
- Online
- Website
Social media
- 2 days
- Online
- Website
HIDA Lecture @ HIDSS4Health
- 1 days
- Online
- Website
Data Science for Supporting Molecular Tumor Boards
Prof. Dr. Ulf Leser, Institute for Computer Science, Humboldt Universität zu Berlin
Molecular Tumor Boards are interdisciplinary teams of medical experts which come together to obtain therapy recommendations for complex oncological cases. In recent years, these recommendations are more and more based on molecular profiling of tumors, in particular the sequencing of cancer genes followed by the detection of genetic variants. The PREDICT project set out to develop systems and algorithms for supporting such decision-making. We will discuss the main results of this project i.e. (1) VIST, a search engine specifically constructed to find clinically relevant documents given a patient’s variant profile, (2) I-VIS, a framework for building integrated precision oncology databases, and (3) BRONCO, the first open and annotated corpus of German medical texts. Building these systems required advanced NLP technologies, the application of modern machine learning methods, and robust data integration technologies.
Ulf Leser is a full professor at the Institute for Computer Science at Humboldt-Universitaet zu Berlin. He obtained his PhD in Data Integration from Technische Universität Berlin. After app. three years in the software industry, he returned to academia as professor for Knowledge Management in Bioinformatics. His main research interests are semantic data integration, text mining, statistical Bioinformatics, and large-scale scientific data analysis. His group not only develops novel algorithms for these tasks but also applies them in a set of highly interdisciplinary collaborative projects for studying concrete biomedical questions, especially in cancer research. He is speaker of the Collaborative Research Center "Foundations of Workflows for Large-Scale Scientific Data Analysis (FONDA)" and of the collaborative research project "Comprehensive Data Integration for Precision Oncology (PREDICT)". From 2014-2019, he was speaker of the Research Training Group "Service-oriented Architectures for Health Care Systems (SOAMED)". Furthermore, he currently is principal investigator in the interdisciplinary graduate schools "Computational Methods for Precision Oncology (CompCancer)“ and “Helmholtz-Einstein International Berlin research school on Data Science“.
Register today!AI-HERO Hackathon for Energy-Efficient AI
- 3 days
- Online
- Website
Managing a Constructive Relationship between PhD Students and their Supervisors
- 1 day
- Online
- Website
Publishing in Scientific Journals
- 3 days
- Heidelberg
- Website
HIDSS4Health Retreat 2022
- 3 days
- Bad Urach
Scientific Writing Course
- 3 days
- Heidelberg
- Website
Six Main Tasks in Image Processing: an Overview
- 1 day
- Online
- Website
Tomographic Methods in Medical Imaging
- 1 day
- Online
- Website
Restoring Noisy Microscopy Images
- 1 day
- Online
- Website
Tracking of Objects: from One to Many
- 1 day
- Online
- Website
Machine Learning for Image Segmentation
- 1 day
- Online
- Website
Visualization
- 1 day
- Online
- Website
Knowledge Representation with the Semantic Web and Knowledge Graphs
- 1 day
- Online
- Website
This lecture aims at introducing the Semantic Web in a nutshell and to show the possibilities it offers:
• An overview of applications of Semantic Web technologies.
• Elements or tools (e.g. RDF/RDFS, OWL, SHACL, JSON-LD, SPARQL) of the Semantic Web stack.
• Use cases how the Semantic Web follows the FAIR principles and can be applied (e.g. Named Entity Recognition (NER), Question Answering, Semantic Search, Expert Systems, Knowledge Discovery)
Join the lecture from 11:00 am to 12:15 pm. Register here!
Explainable Machine Learning
- 1 day
- Online
- Website
Yearly Overview

News

HIDA
As domain scientists from all research fields need to be equipped with knowledge, methods and tools in the areas of Information & Data Science, a training and education initiative for researchers in the Helmholtz Association has been established. The Helmholtz Information & Data Science Academy (HIDA) will coordinate networking, education and training activities throughout the entire Helmholtz Association (video). It bundles the resources of all Information & Data Science Schools (HIDSS), including HIDSS4Health.

Contact
Office Karlsruhe
Karlsruhe, Germany
Phone: +49 721 608-28672
Email: office@hidss4health.de
Office Heidelberg
Heidelberg, Germany
Phone: +49 6221 42-2327
Email: office@hidss4health.de